Eye tracking does not equal attention
When it comes to measuring attention, it can seem that all eyes are on eye tracking. But this narrow focus can be short-sighted, argues Adludio’s Ian Liddicoat.
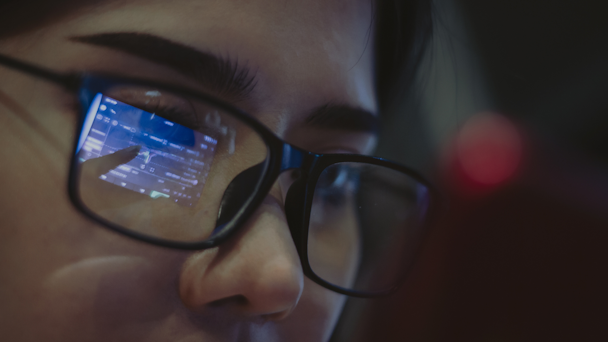
/ Adobe Stock
When professor Byron Sharp questioned the advertising industry’s approach to attention and the metrics around it, he triggered a fierce debate. To an extent, he was right – the industry needs to address the way it views attention. A big part of this is down to how attention is measured, and the tactics employed to drive attention.
Naturally, as third-party cookies and other identifiers disappear and the effectiveness of traditional measurement approaches declines, the industry has been forced into shifting its focus. Attention is definitely the future of measurement, but the way it’s currently measured doesn’t always live up to expectations.
Advertisement
The eyes don’t always tell the full story
One of the most popular methods of measuring attention is through eye tracking studies. While useful in some cases, eye tracking can also result in what could be viewed as implied attention.
Indeed, eye tracking techniques and the corresponding research studies will provide a measure of attention in that the user’s eyes are known to be viewing part of a screen, but that does not guarantee active attention or positive ad recall when tested. There are also some technical shortcomings with some eye tracking, particularly around factors such as certain eye colors, light refraction and whether the individual is wearing glasses or contact lenses.
Now, we’re not here to criticize eye tracking. It has some imperfections but it definitely has its use cases – and particularly for linear TV. Instead, advertisers – specifically digital advertisers – need to consider how attention metrics can be derived from measurable, behavioral data, tuned to their brand and optimized going forward.
A sound measure of attention should be privacy-compliant, sophisticated, data-driven and granular. In turn, these measures should be optimized via machine learning techniques, in order that the relationship between attention and other events, such as click-through, can be clearly understood.
Advertisement
Achieving optimal performance
At the heart of any attention measurement approach should be the consumer and the manner in which they have actually interacted with an ad. This includes asking questions such as, ‘Has the user spent a certain amount of time within the action areas of the ad? Was the sound playing? Have all data points that can be identified been optimized?’ Using data in this way ensures that attention metrics are focused on the quality of that attention and how this drives total campaign performance.
The data derived from these engagement-focused attention metrics can be used by AI to review the creative objects within an ad and measure the quality of its attractiveness in real-time. This insight can then be used to adapt or reassemble the creative to make it as engaging as possible. This, in turn, can inform the underlying ad design processes and ensure that creativity operates in a cycle of performance and innovation.
These insights can also be used to influence algorithmic targeting towards higher attention publishers and inventory. Moreover, if audience data is available, the relationship between creative treatment, sites and inventory can all be optimized to attract a given target group.
Approached in this way, attention becomes both a business goal as well as the target for machine-learned optimization, where all other insights such as creative features are used as inputs to achieve attention thresholds.
Eyes on the prize
There are a lot of techniques out there for measuring different forms of attention or engagement. Some of these are designed to address the ever-growing focus on online data privacy around the world, while others are focused only on one dimension, such as creative features.
The best of these will use customer interactions, apply a range of AI techniques and optimize to an attention metric. Further still, the most powerful solutions will be those that are able to describe how these attention metrics have been derived in terms of the other attributes of a campaign such as the sites, placements, bidding performance as well as creative effects.
Advertisement
This is a logical progression for the digital advertising industry, as the combination of AI techniques and massive computing horsepower begins to demonstrate an ability to significantly improve ad attention and the relationship between this and sales.
The winners will be those who understand how to define clear attention metrics, optimize them and then derive the necessary insight to improve them over time. This will then become a battle for the hearts and minds of consumers and not just their eyeballs.
Ian Liddicoat is chief technology officer and head of data science at Adludio.