Why algorithm calculations need to be transparent following UK exam results
There’s a joke going round that you need a maths GCSE to count the number of u-turns the government has been forced into over the past few weeks following its approach to exam results.
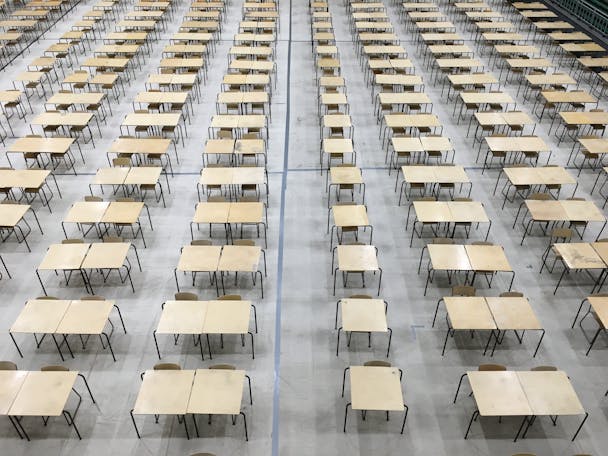
RAPP consider what lessons were learnt from the UK's exam result debate.
The debate surrounding the 'algorithm to predict exam results' saga largely focuses on whether the algorithm was fair, accurate, and systematically unbiased. But what I’d like to explore is what broader lessons can be learned from the whole debacle for those of us who work with consumer-facing algorithms on a daily basis.
Simplicity is often the best option
For the sake of argument, let's assume that the best possible algorithm had been developed that almost perfectly predicted exam results, with no systematic bias, as Ofqual claimed in its report. Imagine you open your exam results after months of nervousness, and your results for a key subject have been downgraded. Without understanding how the algorithm works, you would have no idea why and are bound to be disappointed and angry. Unsurprisingly, the whopping 319 page explainer released by Ofqual required to describe the whole nine-step process, including complex notation to “aid further understanding”, didn’t help matters.
Would a simpler algorithm have been less accurate, and more biased? Perhaps. But what we can say with certainty is that an uninterpretable and unreproducible model would never have gained the confidence of students who missed the grade.
To be fair to Ofqual, they also released ‘standardisation’ reports to schools to explain how they arrived at these grades. It’s just that this seemed to raise more questions than answers.
The lesson here is that, sometimes, a simpler method is preferable to a ‘better’ method. For the method to be accepted by students and teachers alike, it had to be easy to interpret and ideally replicate. It wasn’t.
Be transparent throughout the entire process
There are multiple trade-offs in building an algorithm to predict exam results mentioned in the Ofqual paper. For example, benefit of the doubt vs fairness for students in the past, present, and future.
Is it better to have inaccurately inflated two people’s grades, or to have inaccurately deflated one person’s? The stance from Ofqual is contradictory, claiming that “It was decided to seek to maintain overall qualification standards" whilst also stipulating “there were several decision points which presented the opportunity to give benefit of the doubt to students.”
The lack of clarity on where the balance lies only raises doubts as to the outcome Ofqual was trying to seek.
The lesson here is that it is best to agree in advance what makes a 'good' outcome. Rank different ‘trade-offs’ in importance and decide what scenarios are preferable. Then make sure everyone who needs to know is clear what you have decided and why before you implement it.
Always try to back-test models and share results
Examining what the algorithm results versus actual results would have been had they been applied to last year’s data, and applying that against a variety of use cases would have helped address many issues. Ask where might the algorithm give unexpected results and why; how common will those 'edge cases' be; and what can be agreed on mitigating this upfront.
For me, this should have been in the first paragraph of the Ofqual paper, and if the algorithm was relatively accurate, it would have been an easy PR story. A fair retort to any doubters could have been: “If we had used this algorithm last year, 98% of people would have received the right grade”. Or even better: “The algorithm doesn’t work in the following x cases, so we have ensured all of those students will receive the benefit of the doubt and be given the highest realistic grade they could have attained”.
Backtesting in this way could have helped avoid the chaos, fury and emotional upheaval we’ve seen over the past weeks. Ultimately, it serves as a stark reminder that any consumer-facing algorithm must be transparent and clear for it to be truly trustworthy.
James Addlestone is head of data strategy at RAPP.
Content by The Drum Network member:

RAPP
RAPP is a global, data-driven creative community that builds direct, meaningful and high-value relationships between brands and people. At RAPP, with our unrivalled depth of expertise in first-party data, we’ve been observing and cataloguing real people’s lives for 50 years. In today’s world the balance of power has shifted, and customers are in control, which is why we put people and their preferences at the heart of the brand experience. With a talent base of more than 1,600 professionals in 18 offices, we help brands grow the value of real people by understanding what really matters and creating experiences that are right for real people, with real needs, in real time, creating marketing that matters. Our expertise in data and marketing sciences allows us to deliver our clients actionable human insight - an incredible understanding of genuine motivations, observed transactions and actual interactions. Our process reflects how real people think; we balance the left brain and the right, and we do our best work when we bring Precision and Empathy into balance. Building on our data foundation, RAPP delivers a range of capability across social, digital, customer experience and technology.
Find out more